by: Zank, CEO of Bennett Data Science
80% of our clients come to us with one of three concerns or questions. Here are the reasons, along with how we approach each one and what a typical data science engagement looks like in each case.
Concern 1: “Am I collecting the right (or enough) data?”
I hear this a lot less than I used to, especially since it’s so inexpensive to just store everything possible and sort it out later (sigh).
This is a really valid concern. And as I like to say, you can’t predict someone’s shoe size from knowing their car color. There’s just no way to get from A to B in that case.
However, this one is really another question in disguise. It’s generally meant as, “Can we achieve our data science dreams”…which is invariably followed by a list of predictive capabilities they’d like to have.
We’ve heard this one a lot, and here’s what we generally do: we perform a data assessment, where we spend time understanding your product and business objectives. From there, we help you understand the predictive capabilities of the data you’ve collected.
Don’t have enough data, or what is what you have less than adequate? There’s another element here that we run into a lot called feature engineering. That’s a fancy phrase that describes the process of looking at variables in a different way, say pulling “weekend” vs “week-day” out of a boring timestamp, or combining two variables to create something more descriptive.
This work is very time-intensive and usually requires deep domain knowledge, but is generally worth the effort as it can significantly increase predictive performance.
Concern 2: “We’ve got a product, and we’ve been collecting data but aren’t doing anything ’smart’ with it. Can you help?”
This is my favorite one to hear. It’s really fun for us to get in at the beginning of a project or company, to help do things the right way the first time.
There are lots of intricacies here, such as setting up cloud-based data science infrastructure and connecting to your data stores or helping to build new ones, specific to data science use.
All this work goes on before we produce any plots or shiny dashboards, but it’s well worth it! A solid foundation not only decreases data science development time, but makes for a much happier team; both are essential to be competitive in your space.
Again, this one begins with understanding your product. Then looking carefully at the data your collecting and coming up with a roadmap designed to build predictive elements that maximize product revenue in a personalized manner, across all your users or clients.
Concern 3: “We have a team, but we’re not seeing the ROI we expect from data science.”
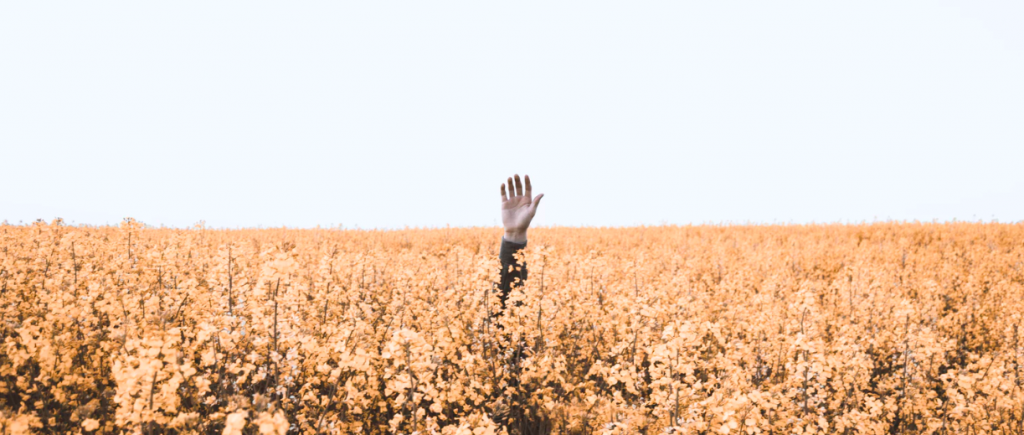
No one wants to admit this one, but some of the most successful leaders we’ve worked with have done just that. Let’s face it, data scientists and data science projects can be challenging to manage for many reasons. Here are some of the common things we see:
- The performance of the team is good but the results are not aligned with product goals
- The team is not producing the desired results and no one knows how to course correct
- The team is leading itself, producing hit-or-miss results, and needs an experienced leader to guide and mentor them
- The boundaries between teams is too blurry and data scientists are working on tasks outside their area of expertise
These are more common than you might think! I’ve seen each of these at large and small companies. The reasons for this can be diverse, from using inefficient tools, to having the wrong team alignment to blockers from supporting technical teams.
Every case is different, but one thing is not; it can always be turned around with the proper support and intervention.
Do you have a question about working with a data science team or making one work more efficiently? Drop me a line or leave a comment. I’d love to hear from you!
Zank Bennett is CEO of Bennett Data Science, a group that works with companies from early-stage startups to the Fortune 500. BDS specializes in working with large volumes of data to solve complex business problems, finding novel ways for companies to grow their products and revenue using data, and maximizing the effectiveness of existing data science personnel. bennettdatascience.com