by: Zank, CEO of Bennett Data Science
Companies build products, and in the process generate tons of data. Data science uses that data to make companies more profitable.
So there it is, in two sentences; in theory anyway. That’s actually a rather optimistic explanation, and not strangely, what our clients expect. The reality, however, is quite different. More on that later.
When people ask me what data science is, that’s generally what I tell them. Notice it’s not a technical explanation. There’s no jargon. There’s no mention of Ph.D.s or complex mathematics. And there’s no mention of infrastructure or methodologies. It’s pure value from data. And that’s exactly what our clients want, ROI. They don’t want cloud-based infrastructure or gradient boosted decision trees. They want to know how to achieve and improve personalization or how to reduce churn. That’s what data science should do. That’s what CEOs want. And that’s what investors want. That’s what we all want. Well, almost.
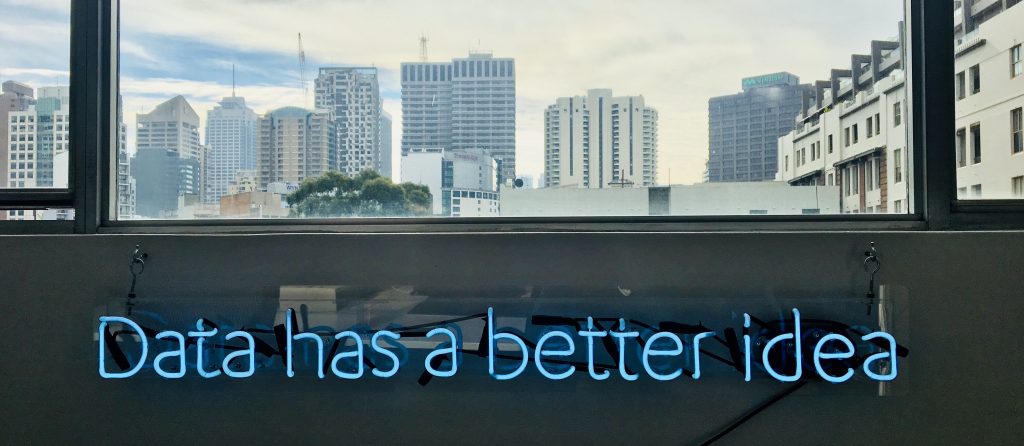
I’ve written a lot about the dire importance of understanding “why” and the objective of data science projects. Those items are critical. They help us answer the question, “When are we done?”. The objective is something that should be easily understandable by a wide audience, most especially the stakeholder guiding the data science initiative. It’s the north star. Follow it until the desired result it achieved. That’s the “Data science uses that data to make companies more profitable” part. Objectives are generally based on KPIs. Data science is just a tool to support the minimization or maximization of those metrics. So when it performs well, or poorly, it should be in terms of metrics that are dead-simple to comprehend. And, they should drive increased revenue (or its proxy).
There’s a great verb that I first heard during my years with the talented team at Trunk Club; “science-ing”. I love this one, and use it any time I can! As it turns out, data scientists love to “science” and will science the heck out of things whenever or wherever the occasion arises. Sounds like a good thing, right? What could be better than a team of smart people science-ing the daylight out of a project? Lots, it often turns out!
Loaded question coming…So, what could possibly go wrong?
Without direction and strong adherence to the “why”, teams can easily get lost. That’s nothing new. But data science has an added trickiness, in that it’s such a difficult field to manage, given the technical acumen that’s required. What we see a lot is a well-intentioned leader managing a team of whip-smart data scientists with little notion of what they’re really working on or even capable of. Data scientists appear busy as ever, churning out plots of metrics from models they’re “working on” and just like that, alignment vanishes. And this type of thing isn’t reserved for small to mid-size companies. It happens at startups to giants like Cisco.
So, what do we do with our two-sentence definition? Should we carefully place asterisks after every other word and footnote it into submission? Of course not. But it’s worth noting that there are two avenues your data science projects can take; on the one hand, with the right team, access to data and leadership, data science initiatives can quickly uncover insights and provide high-value future-looking insights that drive revenue. Alternatively, and far too frequently, they can go over budget and (worse) take much longer than expected.
Zank Bennett is CEO of Bennett Data Science, a group that works with companies from early-stage startups to the Fortune 500. BDS specializes in working with large volumes of data to solve complex business problems, finding novel ways for companies to grow their products and revenue using data, and maximizing the effectiveness of existing data science personnel. bennettdatascience.com