The esteemed journal Nature recently published an article titled, Predicting COVID-19 mortality with electronic medical records.
That’s a big deal. Because it’s not a study looking at predicting mortality from hospital records of admitted patients; rather it uses only electronic health records (EHRs). In other words, if their model is accurate (and it is), it provides a quantitative measure of an individual’s risk. Furthermore, these types of predictive models usually give us a way to understand which factors lead to mortality and which do not. And that is certainly the case in this Nature article.
In this week’s Tech Tuesday I’ll share some of the findings published in Nature’s article and reflect on what these statistics mean when assessing the mortality risks of covid infections.
An Overview
From a 30,000 foot (or, 9,144 meters if that’s your thing) view:
- Age is very important
- Being female protects women slightly
- Previously having pneumonia is a risk factor
- For people aged 45-65, a history of diabetes with complications and cancer (breast and prostate) were notable risk factors
- Those aged 65-85 who have had diseases that affect the pulmonary system are at higher risk
- Overall, there is no evidence that a certain race or ethnicity would alter the chance of mortality from COVID-19, but in the oldest group (85+), being Black was associated with a higher chance of mortality, and being Hispanic or White was associated with a lower risk
All of these findings came exclusively from EHR, making non-invasive risk stratification possible. Using this information, we can now better prioritize who should and should not get the vaccine first. The ease with which the EHR can provide risk makes the process much more scalable than performing some sort of medical assessment.
A bit About the Study:
“We utilized the MGB COVID-19 datamart containing longitudinal medical records from 16,709 COVID-19 patients, to predict risk of mortality and study risk factors for death across different age groups. We demonstrate that the data already routinely collected and stored in EHRs can be rapidly leveraged to address pressing questions related to the COVID-19 pandemic.”
The authors reported the risk of COVID-19 mortality in terms of odds ratios (ORs) for various EHR data. The OR is a simple way of describing the probability that an event will occur. An OR value less than 1.0 indicates decreased occurrence of an event (protective exposure) whereas a value greater than 1.0 indicates increased occurrence of an event.
Factors That Lower the Risk of Mortality due to Covid-19
The OR for mortality in those under 45 years was 0.034. In other words, being younger than 45 years old is associated with a strong protective effect from COVID-19 mortality.
The OR for those between the ages of 45 and 65 was found to be 0.38; much higher, but still below 1.0, so protective against COVID-19 mortality.
Being Hispanic was associated with an OR of 0.31 and being female with 0.65.
Factors That Lower the Risk of Mortality due to Covid-19
Being black was not found to be statistically significant, whereas being identified as White was associated with increased mortality with an OR of 2.45!
And here’s where age comes in. Those between 65–85 years and greater than 85 years were both associated with an increased probability of mortality with an OR of 4.30 and 11.36, respectively.
Of the comorbidities, all increased the probability of dying from COVID-19, but these were found to have the highest OR values: chronic kidney disease, heart failure, abdominal aortic aneurysm, hypertension, and aortic valve disease.
This chart tells the story of the importance of age to predicting COVID-19 mortality:
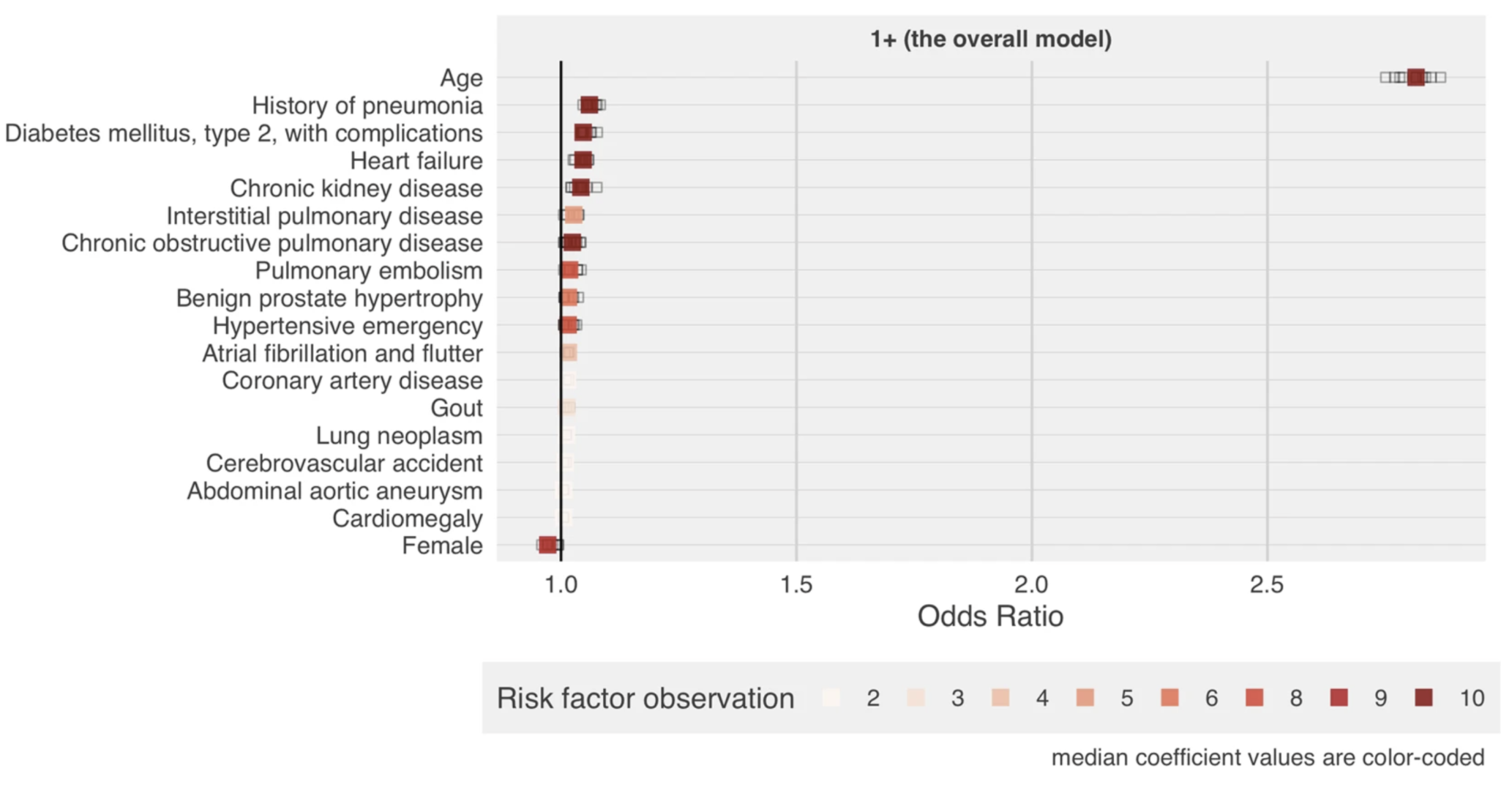
Notice the maroon square (age) in the far upper-right corner? The next highest is “history of pneumonia”, with an OR of 1.06. In other words, pneumonia increases the probability of COVID-19 mortality, but only slightly and becomes even less important when the model is stratified by age, as they did in the paper.
If you have the time and wish to understand what was done, I recommend taking a half-hour to read the journal article; I found their approach appropriate and the write-up informative.
I hope you are healthy! Have a nice week.
-Zank
Of Interest
Where the Latest COVID-19 Models Think We’re Headed
Models predicting the potential spread of the COVID-19 pandemic have become a fixture of American life. Yet each model tells a different story about the loss of life to come, making it hard to know which one is “right.” But COVID-19 models aren’t made to be unquestioned oracles. They’re not trying to tell us one precise future, but rather the range of possibilities given the facts on the ground. Read more here.
The COVID Vaccine Rollout is Leaving Black People Behind
While being black was not found to be statistically significant in terms of when it comes to the mortality risk due Covid-19, black people are certainly unequally impacted. As more and more people are getting vaccinated against the coronavirus, a troubling pattern is emerging: People of color, especially Black people, are being left in the dust. Read more here.
Guitar Machine Learning
If you play electric guitar, you probably know that “tube amps” have been the gold standard since the beginning. Originally, amplifiers were just about making the guitar louder, but it’s become more than that–it’s about manipulating the character of the audio to make it sound more pleasing or achieve some artistic effect. This post focuses on the recent work of leveraging Machine Learning to directly learn the audio processing characteristics of circuits. The authors discuss what it means to digitally model an amplifier and how Machine Learning is beginning to make an impact.