A departure from most weeks, let’s look at something that’s always interested me: how engineers emulate the sounds of classic analog devices, such as keyboards or sound effects, and make them available to anyone with a computer, or even a tablet.
Have you ever wondered how musicians apply reverb to their songs when they do everything on the computer? Where’s the long hall providing all that reverb? Or how a keyboard player on stage (or YouTube these days) can access hundreds of sounds with the push of a button?
The answer is that these sounds (in the case of the keyboard) or effects (in the case of reverb) are digitally emulated. And in this week’s Tech Tuesday, I’ll discuss how these emulations are made.
Digital Emulations
Emulation plugins are made by combining the following two methods:
- By analyzing the electronic circuit and exactly re-creating it digitally; and/or
- For reverb or other effects: sending a series of test tones through the device, or for keyboards or similar: creating sounds with the device and analyzing the output signals as a function of the device settings
The goal is to create a digital emulation that is either indistinguishable from the original device and usually contains options that the original device didn’t have.
Take this EQ audio equalizer for example:

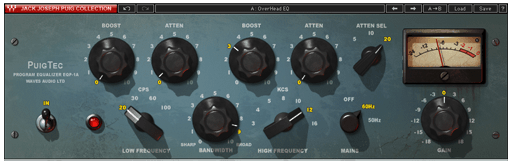
If the reviews are accurate, this plugin sounds identical to the original hardware unit. And with this plugin, a recording engineer can use many instances of it simultaneously for the same cost.
So how do signal processing engineers do it?
Let’s concentrate on EQ for now. In essence, an equalizer (EQ) makes changes to frequencies in music. Too much bass? Use an EQ to subtract away low frequencies, and so on.
To emulate such a device, a known signal is passed into the unit and the deviation from this signal at the output is measured. With the device bypassed, we would expect the input and output signals to be identical. Then, one knob at a time, the settings are changed and the output measured. For complex devices with many knobs and settings, this can be a complex task, to say the least.
And the best part (for the listener) and the worst part (for the engineer creating the emulation) is that a lot of these analog devices deliver nonlinearities that are pleasing to our ears but quite challenging to model with a computer.
Most emulations contain a combination of the two methods mentioned above, since modeling the exact circuit often misses the nonlinearities and warmth that analog devices famously imbue.
Here’s a good article from 2010 and a more recent high-level article on the topic.
Is this something you’ve thought about before? I hope you found it as interesting as I do!
Cheers,
-Zank
Of Interest
Measuring Diversity
Search, ranking, and recommendation systems can help find useful documents in large datasets. However, these datasets reflect the biases of the society in which they were created and the systems risk re-entrenching those biases. For example, if someone who is not a white man searches for “CEO pictures” and sees a page of white men, they may feel that only white men can be CEOs, further perpetuating lack of representation at companies’ executive levels. Using the careful quantification outlined in a recent paper, Diversity and Inclusion Metrics in Subset Selection, we can quantify biases and push these systems to return a wider range of results.
Why is it So Hard to Become a Data-Driven Company?
Thriving as a mainstream company today means being data driven. Companies that have lagged on this front have observed their data-driven competitors seize market share and make inroads into their customer base over the course of the past decade and pioneers like Amazon, Facebook, and Google develop dominant market valuations. Now, mainstream Fortune 1000 companies are fighting back by investing heavily in data and A.I. initiatives to narrow the gap. For the third consecutive year, investment in data and A.I. initiatives has been nearly universal, with 99% of firms reporting investment in data and A.I. This year, however, despite growing investment, it appears most companies are struggling to maintain momentum.
How Data Science is Revolutionizing Our Social Visibility
Artificial Intelligence has the potential to revolutionize the social visibility of brands, paving the way for more incisive approaches towards marketing. This potential has led to Markets and Markets forecasting that the industry of deep learning, machine learning and NLP within sales marketing, customer experience management and predictive risk assessment within social platforms will grow to more than $2.1 billion in value by 2023. The rise of A.I. has been well documented, but how exactly can it enhance your social media marketing strategies? Read more here.